Bike Sharing in Makati City
Exploration of Machine Learning & Deep Learning Algorithms
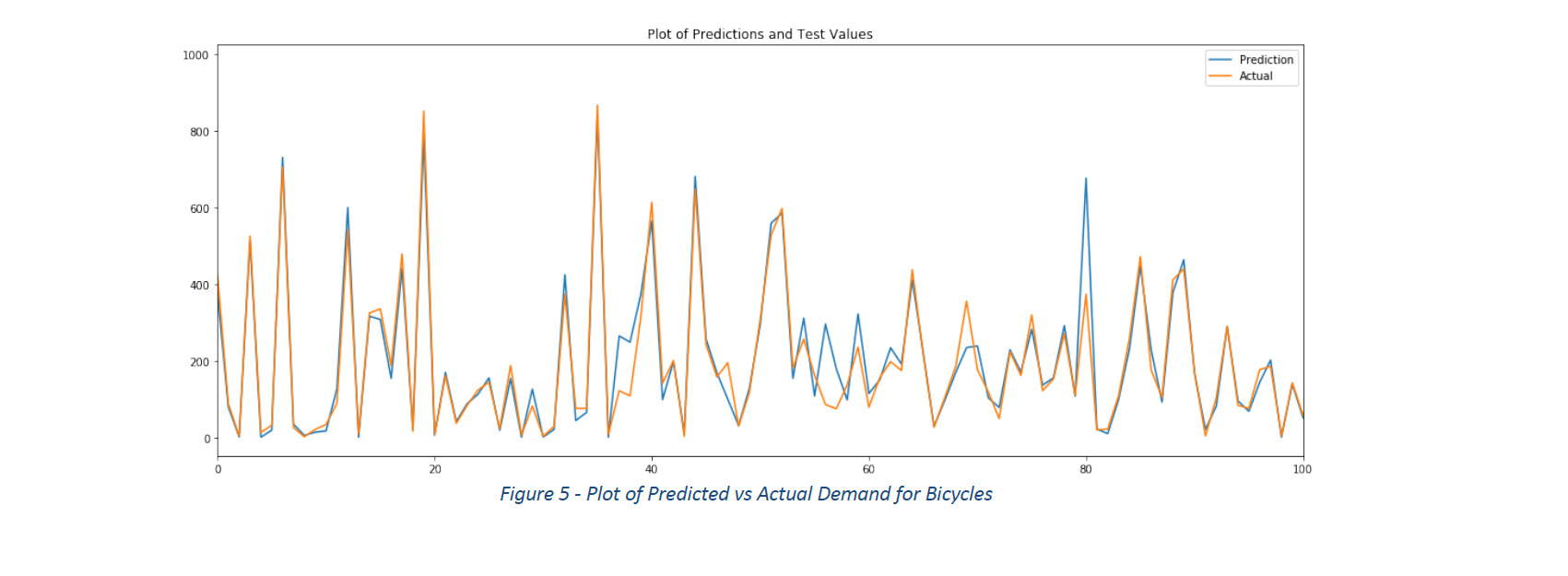
Abstract
To go from one place to another, a person needs to commute either by private car or public transport in order to reach his destination. The problem faced by most individuals, especially those living in Metro Manila, is that it takes too long to arrive in the desired location because of the worsening traffic situation. A minimum of 1 hour and 6 minutes is lost because of the daily commute. One solution to this is to find alternative means of transport that would reduce travel time. Given the limited infrastructure of Metro Manila cities, it would take a considerable amount of time for a transportation project, such as a subway or Rapid Bus system, before it can be completed. One possible stop-gap solution is a public bike-sharing system. This paper explores the possibility of implementing such a project in a pilot city such as Makati City by predicting demand with the use of different Machine learning models trained on the bike sharing dataset of Washington, DC and identifying significant predictors that can be of use to potential stakeholders. The insights and information produced can be utilized by city governments and start-ups for further feasibility and implementation studies.
Introduction
Bike sharing systems are the new generation of traditional bike rentals where whole process from membership, rental, and return back has become automated with the use of sensors. Through these systems, a user is able to easily rent a bike from a particular position with the use of a smart card or a mobile app and return back at another position. Today, there exists great interest in these systems due to their important role in easing traffic congestion and address current environmental and health issues.
Bike-sharing, or public bicycle programs, have seen rapid development and implementation in a lot of cities around the world. With the move of governments towards sustainability and lesser carbon footprints, there have been an increased number of initiatives to enhance bicycle usage, improve the first kilometer/last kilometer connection to other modes of transport, and lessen the environmental impacts of mobility. In 2016 there were already more than 1000 cities with bike sharing systems in place and more than 300 in planning or under construction (Meddin & DeMaio, 2016). This concept was originally from the 1960s, but the growth had been slow until the late 90’s and early 2000’s with the onset of development of better methods of tracking bikes using improved technology. This gave birth to the rapid expansion of bike-sharing programs and startups throughout the Western hemisphere and now most other continents during this decade (DeMaio P. 2009).
Makati City is a candidate for such a program, particularly the Central Business District (CBD). The actual population of the city is only about 582,000 but this swells to over 3.7 million during weekdays because of the influx of workers and shoppers during daytime. The total area covered is approximately 27.3 square kilometers and is the location of the headquarters of numerous international and local companies. It also boasts of several shopping malls and hotels.
Methodology
The following steps were taken for this study:
- Data Exploration and Analysis
- Data Preparation
- Machine Learning Models
- Comparison of Results
If you wish to have a copy of the technical paper for this project, kindly contact us via e-mail or LinkedIn.